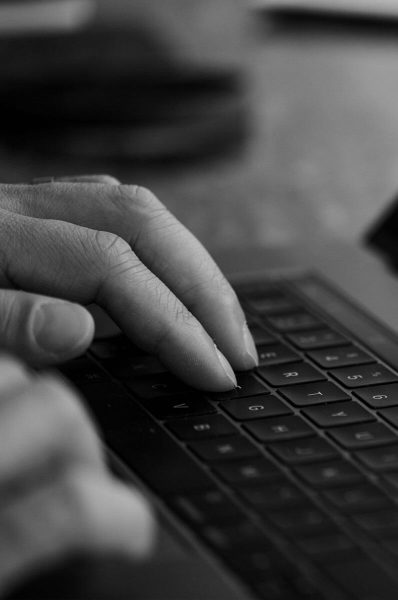
Web Solutions For Any Sized Project
Explore comprehensive web solutions tailored to projects of all sizes. From small businesses to enterprise-level ventures, our expert team delivers scalable and innovative web solutions that encompass design, development, and optimization. Elevate your online presence with custom web solutions built to suit your unique project requirements
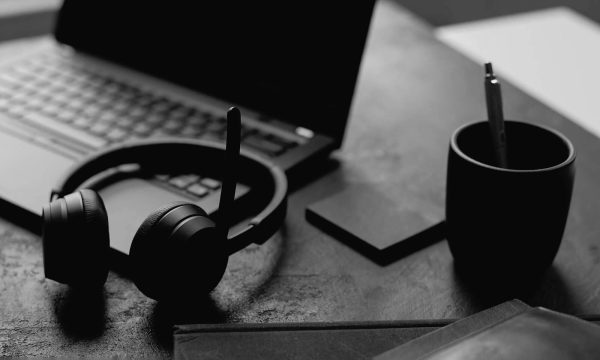
Background
Discover a rich background in providing web solutions for projects of every scale. Our seasoned team brings a wealth of experience to deliver tailored web solutions, whether you’re a startup, SME, or enterprise. From design inception to seamless development, trust our expertise to transform your online vision into a reality.
Education
Educational web solutions designed for projects of all magnitudes. Elevate learning experiences with our comprehensive expertise, catering to diverse educational initiatives. From intuitive platforms for small institutions to robust systems for universities, we craft web solutions that empower education in the digital age.


our expertise in designing and developing user-friendly interfaces ensures
Front End
front-end web solutions
Crafting engaging front-end web solutions for projects of all sizes. Our skilled team specializes in user-centric design and development, ensuring seamless user experiences that captivate and convert. Elevate your digital presence with front-end solutions tailored to your project’s unique needs and goals
Responsive UX Design
Create captivating user experiences across devices with our front-end web solutions. Tailored to projects of any scope, we specialize in designing interfaces that engage and convert.
Scalable Interface Development
From startups to enterprises, our front-end solutions adapt to your project’s growth. Our team crafts flexible interfaces that evolve with your needs, ensuring a seamless user journey.
Mobile Apps
Scalable Mobile Solutions
Whether it’s a startup or an enterprise, our mobile app solutions scale with your project’s growth. We build robust apps that can handle increased user demands and evolving features.
Custom Mobile App Development
Tailored mobile app solutions for projects of any scale. Our team crafts apps that align perfectly with your project’s goals, delivering unique and engaging experiences.
Cross-Platform App Development
Reach a wider audience with cross-platform mobile app solutions. We create apps that work seamlessly on iOS and Android, ensuring consistent functionality and design.
UI/UX Excellence

Custom Development Agencies
WordPress
Self-Hosting with WordPress
With WordPress.org, you have complete control over your website. You’ll need to purchase your own hosting and domain, but you have the freedom to customize your website extensively using themes and plugins.
Managed WordPress Hosting
Providers like Bluehost, SiteGround, WP Engine, and Flywheel offer managed hosting specifically optimized for WordPress. They handle technical aspects like updates, security, and backups.
Domain Management
WordPress.com offers a range of plans, from free to premium, allowing you to create a website without worrying about hosting or domain management.
Let’s Build Something
Let’s Build Something Web Solutions is your partner in crafting digital success. With our innovative approach, we specialize in designing and developing tailored websites that empower businesses and individuals. Our team’s expertise spans from responsive web design to robust e-commerce platforms, ensuring a seamless user experience. Let’s Build Something blends creativity and technology to bring your online vision to life, driving engagement, growth, and meaningful connections
Advantage Technologies
Advantage Technologies is a leading provider of technology solutions, offering a wide range of services and products designed to help businesses and individuals excel in the digital age. Known for its innovative technology and exceptional customer service, Advantage Technologies has become a trusted name in the industry. In this article, we'll explore the company's history, services, team, facilities, customer reviews, community involvement, and future goals. History and Background Founding...
Sentiment Analysis: How Data Science Helps Understand Public Opinion
Introduction Sentiment analysis, a subfield of natural language processing (NLP), leverages data science techniques to understand and analyse public opinion from text data. Data science can contribute to sentiment analysis in several ways. The social and societal implications of data sciences are being recognised widely. The scope of data science cannot be limited to the technology area. For instance, customer retention and customer experience enhancement are areas where sentiment analysis...
Exploring NAS Software: Guide to Network-Attached Storage Solutions
In the digital age, managing and storing vast amounts of data has become a paramount concern for businesses and individuals alike. Network-Attached Storage (NAS) solutions have emerged as a popular and efficient way to store, access, and manage data across networks. NAS software plays a crucial role in enabling these functionalities, offering a range of features and capabilities tailored to diverse storage needs. In this comprehensive guide, we will delve into the world of NAS software,...
The Benefits Of Hosting Your App On The Cloud
Businesses shifting their applications to the Cloud to increase accessibility is no longer a new phenomenon but rather the norm. A cursory glance at the PlayStore or App Store should confirm this. Today, the majority of internet users access emails and websites through their mobile devices. Hosting these applications with the help of traditional VPS or shared hosting environments can be difficult. Hence, the need for Cloud Hosting. Cloud hosting is carried on with the help of cloud servers,...
Tattoo Laser Removal Near Me
Tattoo Laser Removal Near Me in Las Vegas Tattoos have been a popular form of self-expression for centuries, and Las Vegas, known for its vibrant nightlife and entertainment, is no exception. Many residents and tourists in the city choose to get tattoos as a way to commemorate special occasions, express their individuality, or simply as a fashion statement. However, as time passes and circumstances change, some people may find themselves regretting their inked choices. This is where tattoo...
What Are the Benefits of a Dedicated Server To Host Games?
Online games have evolved over the years. According to the statistics, the revenue generated in the Video Games market is US$334.00 billion in 2023, projected to reach US$467.00 billion by 2027. It directly shows the better opportunity for the people and businesses used to hosting games online. However, online game hosting is not as easy as it seems, as it requires high bandwidth and low latency, which helps enhance the user's gaming experience. Therefore, when we talk about Hosting Services...
Beyond Shared Hosting: The Power of cPanel Dedicated Servers
In the fast-paced and dynamic landscape of web hosting, businesses and individuals alike are constantly seeking hosting solutions that offer more power, control, and reliability than traditional shared hosting. The Indian dedicated servers, a robust and versatile hosting solution that goes beyond the limitations of shared hosting. In this blog post, we'll explore the transformative power of cPanel dedicated server and why they are becoming the preferred choice for those who demand more from...
Why VPS is becoming a favourite hosting option for developers
The growth of startups and small- to medium-sized businesses has drawn attention to the web sector. Startups and SMEs are working extremely hard to construct and manage their websites in an effort to increase their business through the acquisition of additional clients through online channels. Because of this, the owners and CEOs of these businesses continue to place the highest premium on having a reliable web hosting service. When choosing a hosting package, these owners may have pestered...
Does Cayenne Pepper Keep Pigeons Away
Introduction Pigeons, often considered urban pests, can be a nuisance in many cities around the world. Las Vegas, known for its vibrant atmosphere and bustling streets, is no exception. Pigeons can cause various problems, including property damage and health hazards. One common question that arises is whether cayenne pepper can effectively deter pigeons. In this comprehensive guide, we will explore the relationship between cayenne pepper and pigeons control in Las Vegas, discussing...